AI Agents: From Tools to Tool-users
Updated: Feb 18
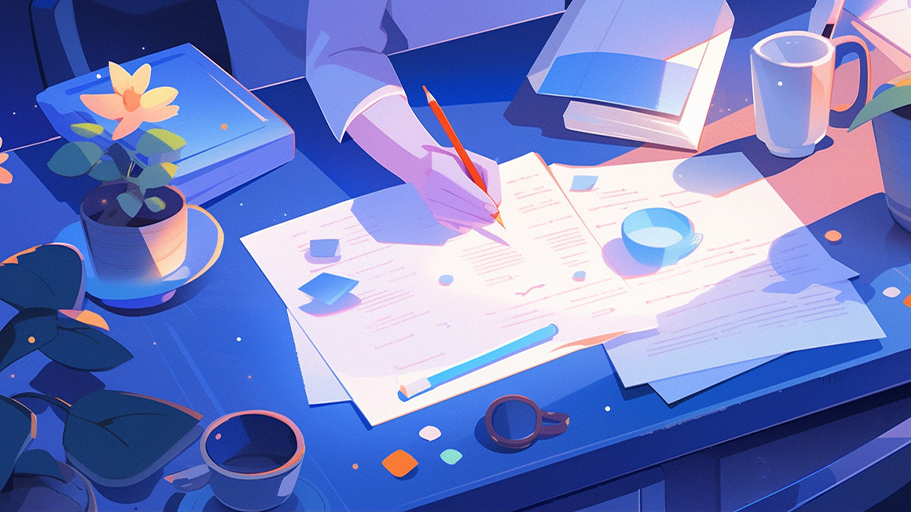
As defined by Wikipedia, A tool is an object that can extend an individual's ability to modify features of the surrounding environment or help them accomplish a particular task. We have seen the evolution of tools, from handmade tools in the Stone Age to mechanical instruments after the Industrial Revolution and Internet software in the Information Age. Now, tools are being rebuilt through AI, displaying unparalleled openness and liveliness than ever before. Recently, you may have noticed a vocabulary shift from AI tools to AI agents. Rather than merely a nomenclature change, this suggests an ontological shift, containing four stages: AI as tools, AI understands tools, AI agents as tool users, and AI agents as tool creators.
AI as tools
AI technology has made significant advancements recently, with more and more software and products incorporating AI capabilities. Search engines like Google and Bing, text-to-image systems like Midjourney and Disco Diffusion, and chatbots like chatGPT and Llama are examples of AI-augmented tools. They are designed to serve specific tasks with AI and are considered instrumental in nature.
The rapid development of AI tools is mainly due to the development of large language models (LLMs), which are trained on large-scale datasets to generate human-like text and perform various language-related tasks. Because of the scope of their parameters, they can generate task-relevant information much more quickly, making them valuable tools for users.
The instrumentality of AI tools is a manifestation of human users’ demand for their functions. People will never refuse a useful helper in their lives. But, instrumentality also means a lack of versatility and autonomy. AI tools can work in a specific area, but can rarely be generalized to other domains. For example, the function of a search engine will be limited to content search. Even if AI broadens the search's scope and optimizes the query results, it will still be limited to a QA domain. Moreover, AI tools cannot autonomously solve problems that require multi-step completion. We never expect Google to organize all the documents, nor do we expect it to bring us a cup of coffee or order a romantic dinner after work. The limitation of AI tools is that they usually only have a single point of ability and cannot comprehensively perceive, reason, plan, and then perform tasks like humans. They do not even understand why they act as they do, and how to act would be more efficient to solve current problems. All they do is follow the instructions of developers/users and follow statistical patterns to perform character combinations that they do not really understand by themselves. Some have pointed out that instrumentality is the most important attribute of AI. The primary goal of human efforts to tackle AI problems is to allow AI to assist in the resolution of problems in human civilization. Therefore, the first step in developing AI technology is to develop problem-solving tools. Some even believed that even if AI technology develops exponentially in the future, we should still limit AI to the status of tools. This can ensure higher security because the final right to action and control still remains in human hands. This demand for instrumentality is still pressing today since LLMs are still doomed to suffer from hallucinations, beset by misleading information, and under some circumstances, even provide damaging information.
AI understands tools
The first step for AI to move beyond instrumentality is to become an entity that can understand. This advancement can be compared to the cognitive development of children. A toddler whose cognitive development is still in its early stages may be able to memorize certain difficult words, but he is not really aware of the meaning behind them. For instance, primary school pupils can memorize the whole advanced algebra textbook if given enough time, but they are unable to comprehend and apply algebraic principles to real-world situations without methodical instruction and a well-developed cognitive system. The AI tools in the first stage are closer to this state of ignorance, like tools without internal instructions. They can only perform specific tasks under the control of users who understand the principles. Nevertheless, AI tools in the second stage can (at least superficially) understand the task. In other words, an AI tool can now understand how it can be used to exert value in a specific task, knowing the internal logic of the task and itself. This is where the capabilities of a simple LLM cannot be achieved, and therefore more modules need to be added, the most important of which is the reasoning part. Researchers have made lots of efforts in this area. Methods like In-Context Learning (ICL), and Chain of Thought (CoT) were proposed to enhance the model's ability to reason, or let's say, to think. Recently, researchers at Deepmind developed a step-back prompting that directs LLMs to abstract higher rules before generating responses, resulting in an improved understanding of the task and its underlying principles.
There is also a special form of reasoning that we call metacognition, which is a higher-order form of cognition that enables AI to reflect on itself. Methods like ReACT and Reflextion are examples of how AI may self-manage and enhance task execution performance, resulting in continually improved understanding. With the aid of various reasoning methods, AI has become a more intelligent tool capable of responding more flexibly to various situations. However, despite this high cognitive capacity, they are nonetheless classified as tools, because they are still task-specific and far from being autonomous entities, which we refer to as AI agents in the context of AI. Being such an autonomous subject requires the addition of new modules, such as memory and action. We can regard the second stage as a transition phase from tool AI to AI agents, but with incomplete architecture. This stage is critical, as the introduction and strengthening of LLM's reasoning ability lies at the heart of the shift from instrumentality to autonomy. Another significant aspect of this stage is that our demand for AI begins to change/upgrade. We are no longer satisfied with AI replacing simple tools but rather expect them to be able to address more complicated and diversified problems. This increased need boosted the development of AI tools and intensified people's desire for AI agents.
AI agents as tool users
After AI can understand the task and understand its own instrumentality, its next step is to apply this understanding to actual actions. At this stage, with the addition of the action module, AI changes from a tool to a tool-user. Lilian Weng has shown how LLM-based agents use tools through systems like MRKL. One practical case is MindOS, the available easy-to-use AI agent-generating platform. On MindOS, users can add [API skills] to let their AI agents (AI Genius) tap into a wide range of platforms, websites, and apps, allowing them to fetch diverse information and interact with multiple services. What's more, users can set up [workflows], arranging and linking components (e.g., Browse Webpage, Google Image Search) together to enable AI agents to execute multi-step tasks.
Furthermore, the action module makes AI agents general across multiple tasks. An AI tool user can call any external tools/resources to solve problems based on an understanding of the current situation. This kind of general AI agent no longer needs to integrate too many functions, but plays value in analysis, selection, and execution. This gives AI agents an economic advantage compared to AI tools. AI agents can get better results with less computation, less data, less manual design, and less post-processing of its outputs.
Except for the economic benefits, AI agents have a more complete cognitive architecture compared with AI tools. Memory is a critical component that provides AI agents with consistency and autonomy across time. With memory, AI agents can remember the preferences and decision-making habits of users in multiple interactions, and autonomously optimize their actions based on these characteristics, leading to more human-like behaviors.
When we discuss memory in daily life, we usually talk about long-term memory, which can be further divided into episodic memory and semantic memory. Here, we will illustrate how memory enables AI agents to possess personalized and professional knowledge with these 2 kinds of memories.
Semantic memory is the memory of general facts. It is the knowledge we gain over our lifetime that demonstrates our expertise. By incorporating such [knowledge] with AI agents, they will be much more professional and excel in credibility. Just like the Industry Analyst and Trip Advisor on MindOS.
Episodic memory, on the other hand, is tightly tied to individual experience and demonstrates personalized features. With episodic memory, AI agents can retain details from previous conversations and neatly organize them, bringing them up at just the right moments, which offers an unparalleled customized experience. And you can do that simply on MindOS with [structured memory].
Just like the important role of memory in human self-identity, memory, especially long-term memory, allows AI agents to have a higher autonomy. They have a past, and therefore can better predict the future and continuously improve themselves on advanced cognitive abilities like planning and reasoning. Such autonomous AI agents may draw ethical concerns. However, with proper design and alignment, AI agents are controllable. They can be seen as supersets of AI tools and can always reduce themselves back to tools whenever needed. AI agents would and should be able to cede their power to human users, providing not only higher but also safer efficiency for mankind.
AI agents as tool creators
Although AI agents as tool users are already intelligent, they can deal with so many problems that AI tools cannot handle. However, a single agent still has a lot of room for improvement, because they are limited not only by the underlying foundation models but also by their domain expertise. While existing tools may not necessarily be able to cope with the growing diverse task scenarios, it is necessary to create new tools rather than just relying on existing ones. This requires agents with different professional knowledge to collaborate, just like human groups cooperate in society. By dividing labor, a group of AI agents can create new tools without excessive human intervention. For example, AI engineers who are good at coding and AI product managers who are good at product development can work together to develop new software to address an unsolved problem. GPTeam and GPT Researcher are good examples of this collaboration, manifesting a deeper connection of AI agents. In this new paradigm, AI agents and humans inspire and learn from each other as close partners.
Therefore, the final form of the AI agent's development is to strip away its instrumentality. In other words, AI agents will not specifically serve to solve problems in the long run, but will gradually become multi-purpose participants in society with motivations and goals just like mankind. Such agents not only play an economic role but also broaden the boundary of human civilization.
Conclusion
One clearly foreseen and important development in the near future is the identity shift of AI agents from tools to tool users and tool creators. MindOS is a fervent supporter of AI agents. On MindOS, you may create various AI agents (named AI geniuses) with professional expertise, customized memory, and sophisticated skills that can help you cope with diverse situations autonomously. Now, you can even create your Personal AI, a special kind of AI agent with more personalized features, to assist you in organizing daily events and tackling more challenging tasks.
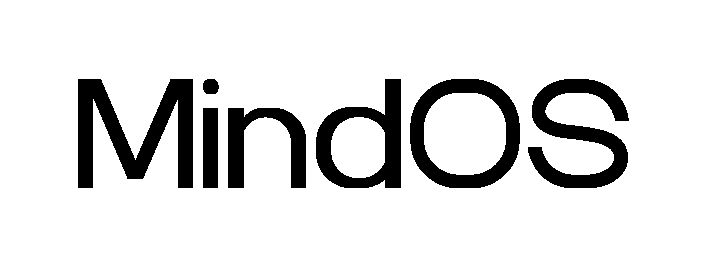