Introduction
In the next few years, we can expect to herald the development of Artificial General Intelligence (AGI) along with all that such a development means for human advancement. With this technology, though, comes a new challenge. With the ubiquity of such tools, there is a real danger of an AI system steering people into creating a single human monoculture.
Here at Mindverse, we want people to never lose what makes them unique.
In recognizing this danger, though, it offers us an opportunity to steer clear of its risks. We have a chance to leverage the infinite possibilities of AI while also designing these systems to embrace what it means to be truly human. Such a system should help people realize their full potential, offering betterment for all people.
The capabilities of tools such as AI are still expanding, though, and are also starting to affect every corner of our lives. As these powerful systems become ubiquitous, the key question emerges:
At Mindverse, we know that technology has the power to understand every person's unique values and perspectives. As such, our aim is not to create a single, universal AI solution. Instead, we want our AI to understand the inherent nature of everyone who uses it so that it can continuously improve what it can provide.
This ambition has driven us to focus on developing the Large Personalization Model (LPM).
Below, we have discussed how we intend to step up to this challenge and what it took to start the journey towards building an LPM. This journey has not been easy, but we know that this innovation sets a new standard in understanding and serving every potential user.
We want to share our experience in developing this system and show how it is becoming a very real and intelligent companion. So, read on to learn more about how it helps you maintain your individuality in an increasingly homogenized world.
Technical Challenges
In building a Large Personalization Model, we knew we would face several challenges before it would meet our expectations. The following details a few of these as well as how we faced them:
Privacy Protection and Data Security
We understand the challenge of privacy protection and data security in 2024. By securing user data and providing clear usage policies, we wanted to give users complete visibility of what we do with their data.
As such, our first challenge in building such a system was protecting the privacy of every user's information. To ensure this, we made sure to:
-
-Use the most advanced data encryption and anonymization technologies available
-
Strictly follow international data protection regulations, such as GDPR
-
Encrypt all information collected through MeBot before it undergoes LPM processing
-
Allow users to define which data is off-limits to the AI
By taking all these steps, the user remains anonymous. This ensures users can trust in the security and confidentiality of their data.
Data Bias and Ethical Modelling
We are very aware that data-driven models risk amplifying existing biases present in training data. To prevent this issue, we took time to redesign our data collection and model training processes. Through this process, we ensured our personalization models treated all user groups in an equitable fashion.
Moving forward, we also intend to conduct regular conduct bias and fairness assessments. This will ensure we continue to optimize our models, responding to potential issues as they start to appear. We will also continue to engage with our audience, especially those from minority user groups who may be the most affected by such issues.
Algorithm Transparency and Interpretability
To make sure our AI systems welcome both users and regulatory bodies, we commit to improving the transparency of our models. This not only means in terms of the data we provide, but also how accessible and understandable such data is.
Despite it being a complex technical challenge, we aim to enable users to understand the decision-making process of our AI models. We can do this by adopting accessible AI technologies and providing a high-quality user experience at every step.
Continuous Learning and Adaptability
From the outset, we designed our personalization model with the ability to adapt to changes in a user's habits over time. However, the complexity of large models and the vast number of parameters make adjustments and updates difficult.
"Transfer learning" adapts to new knowledge slowly and consumes a huge amount of computing resources. To address these issues, we adopted a modular model architecture. Using this method, key components update independently of one another.
Not only does this improve the model's adaptability, but it also reduces the resources the model requires. On top of this, we ensure we always use the latest algorithm optimization techniques. These improve the model's learning efficiency of new information, reducing the amount of waste that occurs.
Performance and Scalability
As the user base and data volume of our system expands, maintaining the system's high performance and scalability is crucial. To ensure this, we have continued to optimize the model architecture we employ and have tracked our computing resource use over time. This has allowed us to respond to processing issues and remain fast when faced with constantly increasing data scales.
Our Technology
We designed the following LPM structure to overcome many of the difficulties we faced during development. The intent of this model is ultimately to achieve a diverse, growth-oriented experience in the AGI era.
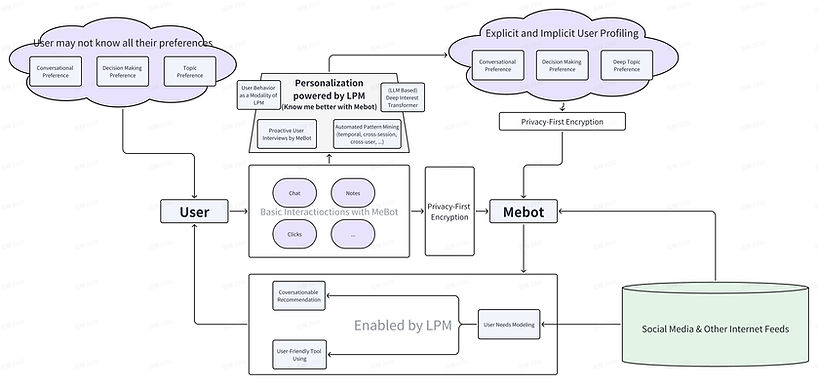
Click to expand image
Understanding and Respecting User Diversity
In building an LPM capable of understanding user diversity in a real and sincere manner, we discovered an unexpected truth:
Many people interact with various places online, but they do not know the amount of information they start to accrue related to:
-
Online messaging and conversation topics
-
E-commerce stores and decision-making habits
-
Search engines and personal interests
On the MeBot platform, we use advanced Large Language Models (LLM). More specifically, we use LLM-Native's deep interest network.
This technology allows us to perform real-time analysis of user interaction data. Over time, this allows us to construct a dynamic, unique user profile.
Furthermore, we've integrated LLM's automated interest mining capability into our system. This allows our LPM to extract non-intrusive data from even minor interactions between users and MeBot. They are then encoded by our Sequential Temporal Network and integrated into our Foundation Model.
Together, all these steps make it easier for us to discover and comprehend implicit user patterns even they may not be aware of. We can then use these to offer the user a far better experience that feels attuned to their daily life.
Continuous Learning and Adaptability
We wanted to support the continuous learning and adaptability of LPM. To this end, we've built it to make use of a dynamic, feedback-driven learning framework.
We leverage advanced AI technologies such as:
-
Real-time model iteration
-
Symbolic Distillation.
Using these, we can finely adjust users' interest models without affecting the core of our Foundation Model. We can also use smaller models at the right time to improve service efficiency without sacrificing response accuracy.
The incremental interest mining mechanism is also an efficient companion to unique user profiles. It adopts a fine-grained update method, allowing our model to continue learning from each interaction with MeBot.
Whenever users interact with MeBot, our system captures new data in real time and integrates it into the user's interest model bit by bit. This method not only reduces the need for large-scale data processing but also keeps user interest profiles up-to-date. This lets us respond to a user or community's latest trends and preferences without throwing up other issues.
Meanwhile, our decay algorithm reduces the priority weight of outdated information in the model over time. This is not a simple time decay process but considers several factors, such as:
-
The frequency of user behavior
-
The type of behavior the user engages in
-
The relevance of the behavior to current trends
Together, these achieve the goal of making sure all interest updates are both dynamic and context-sensitive. This decay mechanism allows our model to develop a unique experience for each user that updates to fit an evolving user.
Personalization and Recommendations in the LLM Era
Based on users' interests and historical thoughts shared with MeBot, LPM will identify users' real needs:
-
"Should I write an email to Desmond now to confirm that yesterday's work has been completed."
-
"The latest US election poll results are out, do you want to see them?
-
"I've organized a timeline for you based on your calendar."
We know that recommendations in the LLM era need to be more conversationable. This puts people at ease, allowing them to take an interest in shaping the recommended content.
When you use MeBot to book hotels, for example, it will tend to book familiar brands, room types, floors, and so on. This is due to the interests and usage habits it learns over time.
Try the Large Personalization Model For Yourself
We know this is a lot of information, so you may want to check out our LPM for yourself. Gone are the days of stale, impersonal recommendations. Instead, once MeBot learns who you are, you will never need to worry about poor-quality information again.
If that sounds like the kind of thing that would help you, then now is the time to try Mebot for free and find out what it can do for you.